FX Volatility Surface Construction:A Comprehensive Framework for Analyzing FX Volatility Surface Dynamics
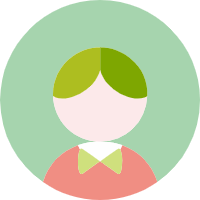
The foreign exchange (FX) market is a complex and dynamic environment, characterized by high volatility and uncertainty. As a result, understanding and managing the risk associated with foreign exchange transactions has become increasingly important for investors and businesses. One of the key risk factors in the FX market is volatility, which refers to the likelihood of price changes in the future. To effectively manage this risk, it is crucial to have a robust and accurate representation of the FX volatility surface, which is the graphical representation of the expected volatility of currency pairs at various future times. This article aims to provide a comprehensive framework for constructing the FX volatility surface and analyzing its dynamics, with a focus on the implications for risk management and investment strategies.
FX Volatility Surface Construction: A Comprehensive Framework
The construction of the FX volatility surface involves several steps, including the estimation of volatility parameters, the selection of appropriate models, and the integration of historical and real-time data. The following sections will discuss each of these components in detail.
1. Estimation of Volatility Parameters
The first step in constructing the FX volatility surface is to estimate the volatility parameters for each currency pair. These parameters can be obtained through various methods, such as historical volatility, forward volatility, or model-based volatility. Historical volatility is the typical method used to estimate volatility, as it is based on the past price movements of the currency pair. However, historical volatility may not accurately represent future volatility, especially in high-volatility environments. Forward volatility, on the other hand, is based on the future price movements of the currency pair and is more relevant for risk management purposes. Finally, model-based volatility uses mathematical models to predict future volatility, which can be more accurate but requires significant computational power.
2. Selection of Appropriate Models
Once the volatility parameters have been estimated, it is essential to select an appropriate model for the FX volatility surface. There are several different types of models that can be used, such as Gaussian processes, neural networks, and random forest models. Each model has its own advantages and disadvantages, and the choice of model should be based on the specific needs of the investor or business. For example, Gaussian processes are well-suited for high-dimensional data and have a limited number of free parameters, making them suitable for dynamic volatility surfaces. Neural networks, on the other hand, are capable of learning non-linear relationships and can handle more complex volatility surfaces, but may require more time and resources to train. Random forest models are popular for their accuracy and stability, and can handle large volumes of data, but may have a higher computational cost.
3. Integration of Historical and Real-time Data
In order to construct a comprehensive FX volatility surface, it is essential to integrate historical and real-time data. Historical data can provide valuable insights into the past behavior of the FX market, while real-time data can help to reflect current market conditions. By combining these two types of data, it is possible to create a more accurate and robust representation of the volatility surface.
Analysis of FX Volatility Surface Dynamics
Once the FX volatility surface has been constructed, it is crucial to analyze its dynamics in order to better understand and manage risk. Some key aspects of volatility surface dynamics to consider include:
1. Time-varying Volatility: Volatility may change over time, especially in high-volatility environments. By incorporating time-varying volatility into the volatility surface, it is possible to better capture the dynamic nature of the FX market and make more informed investment decisions.
2. Non-linear Relationships: Volatility surfaces may exhibit non-linear relationships, meaning that the relationship between currency pairs may change over time. By using non-linear models, it is possible to capture these complex relationships and improve the accuracy of the volatility surface.
3. Time-series Autocorrelation: Volatility surfaces may have time-series autocorrelation, meaning that the volatility of one currency pair is affected by the volatility of other currency pairs. By accounting for this autocorrelation, it is possible to better understand the interdependence of the FX market and make more informed investment decisions.
The construction and analysis of the FX volatility surface is a crucial aspect of risk management and investment strategy in the FX market. By using a comprehensive framework that includes the estimation of volatility parameters, the selection of appropriate models, and the integration of historical and real-time data, it is possible to create a more accurate and robust representation of the volatility surface. Additionally, analyzing the dynamics of the volatility surface can provide valuable insights into the dynamic nature of the FX market and help to make more informed investment decisions. As the FX market continues to evolve and become more complex, it is essential for investors and businesses to have a deep understanding of the volatility surface and its dynamics in order to effectively manage risk and maximize returns.