The formula for volatility:A Mathematical Framework for Volatility Estimation and Forecasting
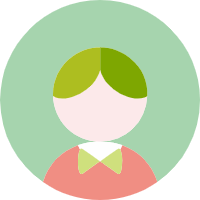
The Formula for Volatility: A Mathematical Framework for Volatility Estimation and Forecasting
Volatility is a crucial concept in finance and economics, as it serves as a measure of the uncertainty in future prices of financial assets. Understanding and predicting volatility is essential for making informed investment decisions, risk management, and economic forecasting. This article aims to provide a comprehensive overview of the mathematical framework for volatility estimation and forecasting, focusing on the relationship between volatility and other financial variables. We will explore the various approaches to volatility estimation and forecasting, their limitations, and potential solutions.
Volatility Measurements
Volatility is typically measured using historical prices of financial assets, such as stocks, bonds, and derivatives. The most common volatility measures include the average annualized volatility (AV), the average daily volatility (ADV), and the standard deviation of price changes (SD). Each of these measures has its advantages and disadvantages, and a combination of measures may be required to obtain a complete understanding of volatility.
Mathematical Frameworks for Volatility Estimation and Forecasting
There are several mathematical frameworks for volatility estimation and forecasting, each based on different assumptions and methods. We will discuss three main frameworks: the arithmetic mean regression model (AMR), the GARCH model, and the machine learning approach.
1. Arithmetic Mean Regression Model (AMR)
The AMR framework assumes that the volatility of future prices follows an arithmetic trend, with the volatility increasing or decreasing at a constant rate. This model can be represented by the following equation:
Volatility_t = Volatility_t-1 + λ_t
where Volatility_t is the volatility at time t, Volatility_t-1 is the volatility at time t-1, λ_t is the volatility trend, and t denotes the time index. This model is simple and can be used for volatility estimation, but it may not capture the complex patterns of volatility in financial markets.
2. Generalized Autoregressive Conditional Heteroskedasticity (GARCH) Model
The GARCH model, proposed by Bollerslev in 1990, is a more complex framework that considers the heteroskedasticity of returns, meaning that the variance of returns changes over time. The GARCH model is represented by the following equation:
Variance_t = ∑_i β_i * (returns_i)^2 + γ_t * (Variance_t-1)
where Variance_t is the variance at time t, β_i are the coefficients of the autoregressive term, γ_t is the coefficient of the conditional heteroskedasticity term, and returns_i are the returns at time t-1. This model provides a more accurate estimation of volatility, but it requires more data and computational resources.
3. Machine Learning Approach
The machine learning approach, particularly deep learning techniques such as recurrent neural networks (RNNs) and long short-term memory (LSTM) networks, has gained popularity in recent years for volatility estimation and forecasting. These methods use large amounts of historical data and adaptive models to capture the complex patterns of volatility in financial markets. However, the accuracy of these methods depends on the quality of the data and the model's capacity to learn from the data.
Limitations and Future Directions
While the aforementioned frameworks provide valuable insights into volatility estimation and forecasting, they each have limitations. The AMR framework may not capture the complex patterns of volatility, while the GARCH model requires more data and computational resources. The machine learning approach requires large amounts of data and adaptive models, but its accuracy depends on the quality of the data and the model's capacity to learn from the data.
Future research should focus on developing more accurate and efficient frameworks for volatility estimation and forecasting, taking into account the complexity of financial markets and the various measures of volatility. Combining multiple frameworks and methods may provide a more comprehensive understanding of volatility and improve the accuracy of volatility forecasting.